What is NLP? Future of the Translation and Artificial Intelligence
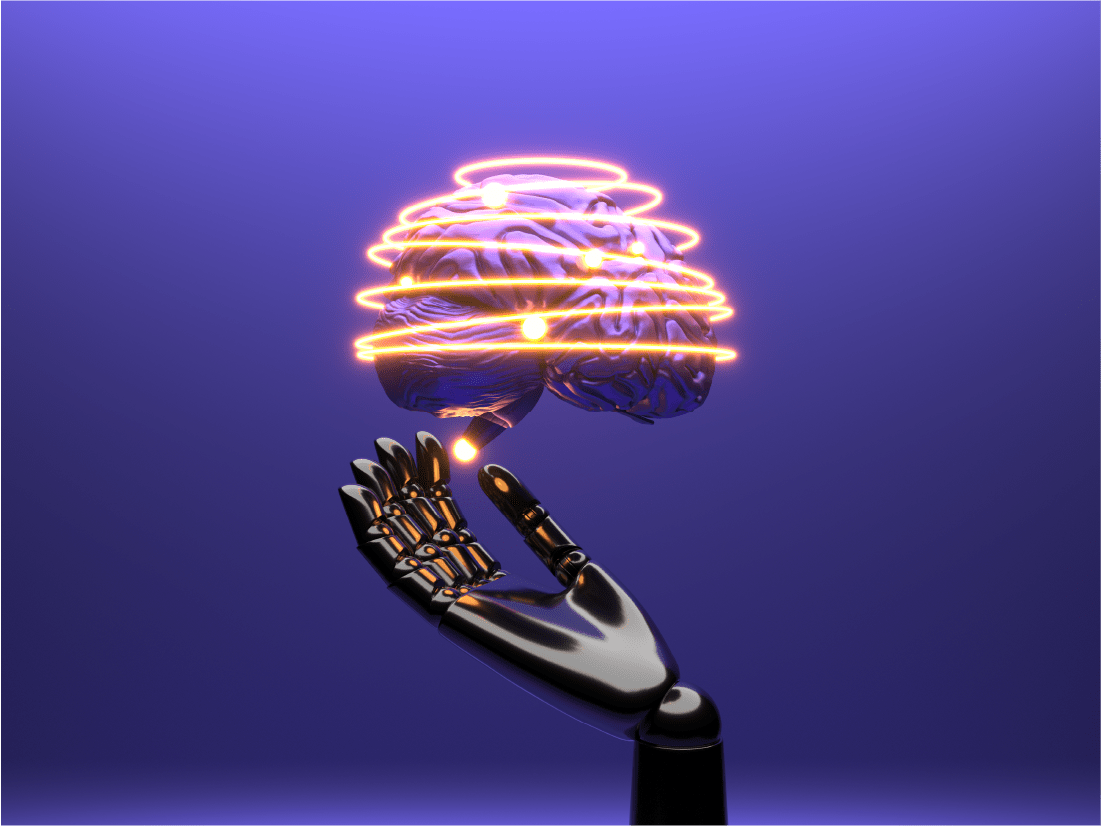
From the pen of Berk Yazar, one of our Digital Translation and Localization Institute 2022 graduates
Natural Language Processing (NLP) is an interdisciplinary field rooted in artificial intelligence and linguistics. Starting by laying the base of artificial intelligence in the 1950s, this long journey has witnessed huge breakthroughs in many fields which affect people’s daily lives, such as medicine, communication, trade, and transportation, since 2010. Because of the increased needs, the language industry has also started to get shaped around the light of this technology and lots of natural languages (NL) based subfields have emerged.
The purpose of this paper is to inform translators about the processability and imitableness of natural language by artificial intelligence, basically to explain the working principle of artificial intelligence on human language, to consider this case from the perspective of translation, and to present an approach about which point this technology will reach in the near future with the data we have.
Natural Language Processing technology, which we expect to come across derivatives of in the upcoming years, seems like it will keep the scientists from these two disciplines busy because of the demand that will increase with the growing human circulation, especially after the pandemic and the technological improvement. The natural language-based fields that got diversified with the point of Natural Language Understanding (NLU) and in fact, Natural Language Generation (NLG) in today’s world, which artificial intelligence aims to get to, are of course, directly connected. Well then, how did the carried-on studies about the algorithm that was present at the root of machine learning and language-based artificial intelligence studies start?
Technological pioneers such as Google, and Microsoft have been carrying out studies about artificial intelligent natural language learning for many years. If we compare these studies to a human and look at this example, it may be possible to understand the working principle of artificial intelligence and make it more recallable. A person instinctively collects data from their surroundings about the culture and language of the region they were born in from the moment of their birth and thus, learns to speak in a couple of years by imitating the sounds they hear and gestures they see. In other words, they try to verbally communicate first. Artificial intelligence also emerged by imitating human. It is again a human that controls the artificial intelligence; therefore, it follows a path similar to one that a human follows in language learning. That is why our first step in the natural language learning is to look at examples of sounds; among these examples, the most striking ones are Text-to-Speech and Speech-to-Text technologies.
At the heart of this technology, there lies an effort to accurately translate an utterance formed with millions of combinations and uttered through sounds in thousands of languages into a different sign in the least amount of time. This was not technically possible before the artificial intelligence. If there are too many data points and too many decision-making processes, in other words, if we are talking about data points and processes that are too big to do manually or with processors, it was a necessity to adapt the artificial intelligence according to language so as to make what was wanted possible. For example, let’s assume there are 10 different audio files with digital data saved in the file, that we know what is inside. There is one letter in each of these files, and it is necessary to find which file has which letter while the program is still working. We aim for the artificial intelligence to utter the different two files, “s” and “a”, as if it were “sa” like a human would, instead of uttering them separately if these letters are collocated. In this respect, artificial intelligence tries to find a similarity by scanning all the file contents, analyzing other samples where the letter “s” was matched before among the files in the database, comparing one by one, processing, and analyzing, and deciding whether the pronunciation of the letter recorded in the file is “s” or not. Artificial intelligence repeats this process to accurately utter every single letter in utterances. Even in an alphabet of 10 letters, it needs to try all the combinations; however, at this exact moment, deviating from the main purpose occurs because it is a complicated process it is not possible to give a quick output. Following this process during the time the program works means it will take a while. To do the calculation quickly or to present output without calculation, all the other samples need to be calculated before; therefore, artificial intelligence can find the file and decide quickly. For this reason, all the files are saved on the artificial intelligence board in a mixed way.
The second significant element in Natural Language Processing is the tolerance function, bias, which decides whether the letter in the file is “s” by putting the sound waves on top of one another. The working principle of the function is generally to test all the files and understand if the file has the letter “s” in it or not. The files are tested and saved. When encountering new data, looking at the frequency, amplitude, and length of the audio file that will be compared, it reaches the related data, and thus, it puts forth an output by presenting only the confirmed file in the related data. And says it detected the letter “s”. When the most difficult step, “detection” is finished, artificial intelligence transforms this data into text or picture according to what the user wishes. As explained simply before, this process has been practiced from the day computers started to be used commonly till today.
Speech-to-Text and Text-to-Speech technologies are the first steps of the NLP process. Human brain also learns language by following a similar path. How does this system work in other fields, then? How are utterances, texts, and expressions that are entered in the program via audio or writing phrased in a different language after following the process as detailed before? In its most general definition, translation is the work of producing different material by looking at a source. When we look at the perspective that translation studies have provided us in the last couple years, it is not possible to approach translation independently of culture. In short, knowing both languages is not enough to translate. In the translation process, where different dynamics are in question, is it possible for artificial intelligence to get past this “culture problem” that is present for itself? For instance, can artificial intelligence localize? Will it be an assistant for the translator or an opponent by processing the natural language humans speak?
Today, artificial intelligence provides subsidiary tools for translators; it helps the translator do the translations that are too big for the translator in a short amount of time with high accuracy. And it does this via two separate elements: the first one is the bias function, as explained above, and the second one is present in the database, providing the machine with equivalences in the source and target languages as translation. A translation program associating the word “I” registered in the English cluster with “Ben” in the Turkish cluster can be given as an example for the second element. In today’s technology, it is possible to expand the example to include meaning in the word, meaning in the sentence, and meaning in the text. What is impossible at this point is for artificial intelligence to deliver the message conveyed in the source text without human intervention and accurately in any circumstances to the target language. Translating simple commands in widely used language pairs would not cause any complexity; however, it is not possible for the machine to translate every expression perfectly with the help of artificial intelligence but not of humans, to express that message according to the norms of the target culture, and to localize under today’s conditions. Therefore, pre-editing and post-editing concepts are essential in translator’s lives. NLP and derivative technologies benefit the translator in that they reduce the time spent on the translation. Apart from helping the translator, they are used in various fields like emotion analysis, analysis to detect target audience preferences, intelligent assistance used in daily life (Siri, etc.), market reports used for globalization, BERT systems developed by Google that aim to get accurate results in accordance with language and culture, discourse analysis, and chat robots limited to certain functions. Natural Language Processing will start to give more organic and accurate results as it keeps being fed with data. Nevertheless, artificial intelligence is a field that has a simple base, yet it takes a long time to work on it, and as long as it is limited, it can give accurate results. The reason Google Translate cannot give accurate results most of the time is that the entry of the data into the system is much faster than processing the data and using it in other translations. For this reason, the translator that detects well the advantages that artificial intelligence provides, knows how to benefit from these advantages, is able to look from a wide perspective, and, in short, “looks at one source, and is able to present a different product in accordance with the demands” will be the translator that survives in the market even if their title changes…